Data science has always been a powerful and fascinating discipline for anyone working in media, but it’s about to get a whole lot more interesting. That’s thanks to pioneering advancements harnessing large-scale data sets from diverse sources such as connected TVs, service operators, and online platforms. Kantar Media has been a pioneer as the first measurement company to build an in-house Data Science team and with these new advancements the team is now exploring new possibilities to provide enriched measurements of audience viewing.
The rise of connected TVs (CTVs), for example, is transforming media consumption while offering an abundance of data for deeper insights into audience preferences and behaviours. This rich data equips media companies and advertisers with a comprehensive audience understanding, enabling targeted content recommendations, optimised advertising campaigns, and effective marketing strategies. It’s an important trend underscored by major businesses like Comcast, Roku, and Charter investing in their own TV sets, highlighting the significance of CTVs as a critical data source.
However, as data sources (and media choices) expand, this presents us with some challenges, such as audience fragmentation. This is where data science is assisting - by integrating large-scale data from connected TVs alongside panel demographic data, enhancing the granularity of insights. Think of it as a way of strengthening the signal.
Kantar Media is employing data science methodologies, allowing for the assimilation of panel demographic datasets, with a portion of CTV data broadening the scope of the sample. In turn, this fortifies the depth and reliability of the insights, and improves the granularity of the data as evidenced by Kantar Media’s work in Norway and Finland over the last five years.
For instance, a recent trial with the Spanish children's broadcaster CLAN (RTVE) leveraged a segment of CTV census data to expand its sample size to 100,000 individuals, which markedly refined the data granularity and yielded more consistent daily audience profiles, removing zero ratings almost entirely (see chart, below). Following the data fusion, the viewership pattern becomes more uniform while still maintaining the established trends.
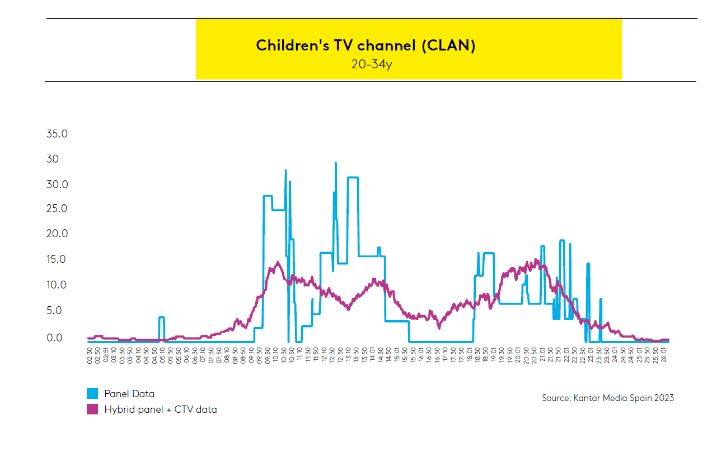
Although the benefits are enormous, harmonising data from diverse sources into a single dataset presents a host of complexities and challenges. Each data source - be it from linear TV viewership, OTT platforms, digital media, or social interactions - operates on its unique metrics and dimensions. The intricacies involved in standardising these varying data points to create a unified dataset are considerable.
This standardisation requires meticulous mapping of different data structures, aligning of measurement metrics, and reconciliation of differing data collection methods. Moreover, the temporal aspect adds to the complexity; data must not only be harmonised across platforms but also across time frames.
The process of unification also involves overcoming technical disparities, such as varying data formats and the potential incompatibility between systems. Disparate data sources often come with their proprietary formats and structures, which necessitates the use of sophisticated data transformation tools and processes to integrate them into a single coherent framework.
Privacy considerations are also paramount, as data from different sources may have varying levels of sensitivity and be subject to different regulatory requirements. Ensuring compliance while still achieving a level of data granularity useful for analysis is a delicate balancing act, although the advent of new privacy-enhancing technologies pave the way to unlock new insights without the need for intrusive individual tracking. .
Strong data partnerships are therefore essential for a comprehensive measurement of VOD consumption. These partnerships allow for the flow of viewership data from platforms to measurement companies, enabling a more accurate representation of what content is being consumed, when, and on what device.
Such partnerships must be built on a foundation of trust and mutual benefit, and oftentimes require extensive negotiations and agreements on data usage rights and privacy protections. But it’s all worth it. For advertisers and media companies, these partnerships ultimately offer an unprecedented understanding of viewing habits, particularly for AVOD and FAST services, where traditional measurement methodologies may fall short.
As Kantar Media's latest Media Trends & Predictions report highlights, the future holds promise for more refined data strategies. Businesses are expected to increasingly utilise predictive modelling, AI-driven data enhancement, and diversified data processes. These approaches will help organisations become more proactive and adaptive, improving both content creation and advertising strategies.
However, despite the wealth of data available and our growing abilities, many businesses fail to make the most of it. This under-exploitation is often due to several barriers such as lack of time, insufficient resources and skills, or concerns about privacy and security. Consequently, the data sits idle, and its potential for driving business growth remains largely untapped.
Moreover, while the benefits are vast, challenges such as data overload, privacy concerns, and data quality issues also exist. So as we sharpen strategies to leverage data at scale, it will be important to take a balanced approach, advocating for both the exploitation of data's potential and the mindful management of its associated risks. Ultimately there is more to be excited about - and I’m thrilled to be in a position to help businesses make the most of it.
Sushmita Jain is Product Director Data Science, Kantar Media
The rise of connected TVs (CTVs), for example, is transforming media consumption while offering an abundance of data for deeper insights into audience preferences and behaviours. This rich data equips media companies and advertisers with a comprehensive audience understanding, enabling targeted content recommendations, optimised advertising campaigns, and effective marketing strategies. It’s an important trend underscored by major businesses like Comcast, Roku, and Charter investing in their own TV sets, highlighting the significance of CTVs as a critical data source.
However, as data sources (and media choices) expand, this presents us with some challenges, such as audience fragmentation. This is where data science is assisting - by integrating large-scale data from connected TVs alongside panel demographic data, enhancing the granularity of insights. Think of it as a way of strengthening the signal.
Kantar Media is employing data science methodologies, allowing for the assimilation of panel demographic datasets, with a portion of CTV data broadening the scope of the sample. In turn, this fortifies the depth and reliability of the insights, and improves the granularity of the data as evidenced by Kantar Media’s work in Norway and Finland over the last five years.
For instance, a recent trial with the Spanish children's broadcaster CLAN (RTVE) leveraged a segment of CTV census data to expand its sample size to 100,000 individuals, which markedly refined the data granularity and yielded more consistent daily audience profiles, removing zero ratings almost entirely (see chart, below). Following the data fusion, the viewership pattern becomes more uniform while still maintaining the established trends.
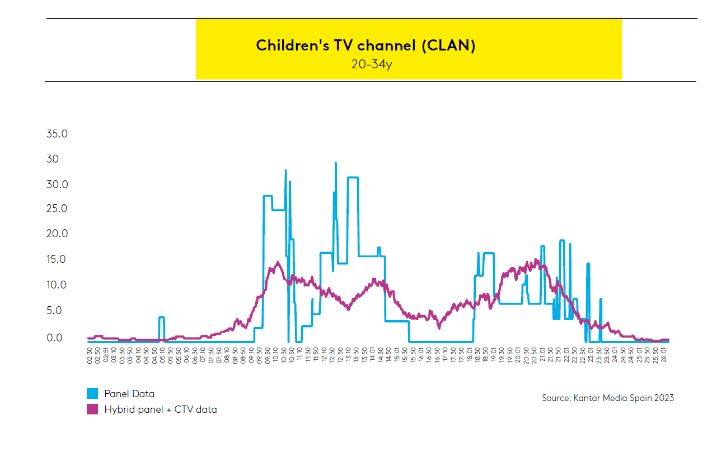
Challenges and complexities
Although the benefits are enormous, harmonising data from diverse sources into a single dataset presents a host of complexities and challenges. Each data source - be it from linear TV viewership, OTT platforms, digital media, or social interactions - operates on its unique metrics and dimensions. The intricacies involved in standardising these varying data points to create a unified dataset are considerable.
This standardisation requires meticulous mapping of different data structures, aligning of measurement metrics, and reconciliation of differing data collection methods. Moreover, the temporal aspect adds to the complexity; data must not only be harmonised across platforms but also across time frames.
The process of unification also involves overcoming technical disparities, such as varying data formats and the potential incompatibility between systems. Disparate data sources often come with their proprietary formats and structures, which necessitates the use of sophisticated data transformation tools and processes to integrate them into a single coherent framework.
Privacy considerations are also paramount, as data from different sources may have varying levels of sensitivity and be subject to different regulatory requirements. Ensuring compliance while still achieving a level of data granularity useful for analysis is a delicate balancing act, although the advent of new privacy-enhancing technologies pave the way to unlock new insights without the need for intrusive individual tracking. .
Strong data partnerships are therefore essential for a comprehensive measurement of VOD consumption. These partnerships allow for the flow of viewership data from platforms to measurement companies, enabling a more accurate representation of what content is being consumed, when, and on what device.
Such partnerships must be built on a foundation of trust and mutual benefit, and oftentimes require extensive negotiations and agreements on data usage rights and privacy protections. But it’s all worth it. For advertisers and media companies, these partnerships ultimately offer an unprecedented understanding of viewing habits, particularly for AVOD and FAST services, where traditional measurement methodologies may fall short.
What’s next?
As Kantar Media's latest Media Trends & Predictions report highlights, the future holds promise for more refined data strategies. Businesses are expected to increasingly utilise predictive modelling, AI-driven data enhancement, and diversified data processes. These approaches will help organisations become more proactive and adaptive, improving both content creation and advertising strategies.
However, despite the wealth of data available and our growing abilities, many businesses fail to make the most of it. This under-exploitation is often due to several barriers such as lack of time, insufficient resources and skills, or concerns about privacy and security. Consequently, the data sits idle, and its potential for driving business growth remains largely untapped.
Moreover, while the benefits are vast, challenges such as data overload, privacy concerns, and data quality issues also exist. So as we sharpen strategies to leverage data at scale, it will be important to take a balanced approach, advocating for both the exploitation of data's potential and the mindful management of its associated risks. Ultimately there is more to be excited about - and I’m thrilled to be in a position to help businesses make the most of it.
Sushmita Jain is Product Director Data Science, Kantar Media